Quantitative finance involves the mathematical modeling of financial markets and securities.
Quantitative trading is a subset of this area that covers all aspects of using mathematical methods in trading markets.
Many pioneers of quantitative trading started with pen and paper, looking for patterns in price actions and predicting moves on the back of this.
But in today's world, quantitative trading generally means using computers to perform the necessary calculations, though strictly speaking this is not always necessary.
If you want to understand what quantitative trading is, here are some key areas you should be aware of:
- Sell side firms
- Buy side firms
- Algorithmic trading
- Arbitrage and HFT
- Data and machine learning
- Risk management
- Portfolio construction
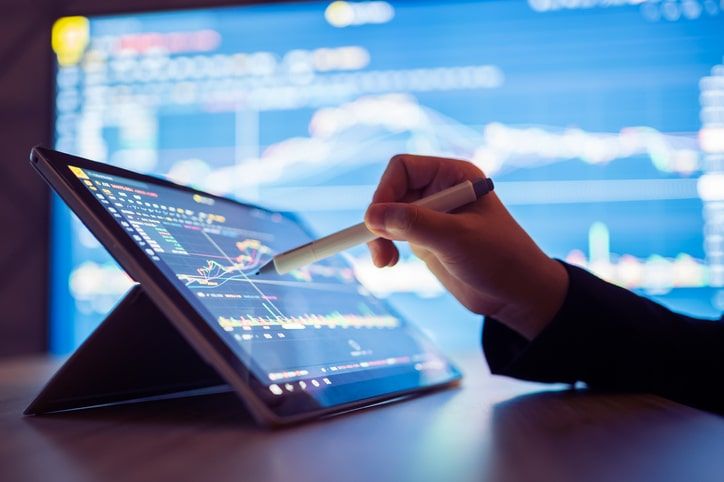
Sell-side firms are primarily concerned with attracting as much deal flow as possible through making prices to their clients.
To that end, the majority of these firms' quantitative efforts relate to improving client access and assessing market liquidity to best differentiate good flow from predatory flow.
The techniques used for sell-side market-making are therefore less mathematical and more technological.
The technological challenges the sell-side faces are in building "pipes and plumbing" that link clients to the market rather than building advanced models for financial engineering purposes.
The revenue-generating model of buy-side firms is very different to that of the sell-side.
Sell-side firms aim to attract deal flow through their books in order to generate income from spreads and commissions.
Buy-side firms, by contrast, generate revenue from taking positions in assets and the subsequent movements in the prices of those assets.
As a result, buy-side firms have very different quantitative trading requirements.
Buy-side firms use quantitative techniques to generate trading signals, which are used to decide which assets to buy and when to buy those assets.
In order to apply these signals to pools of capital, trades have to be executed in the market.
This in itself is a highly quantitative process using execution algorithms that need to consider the impact of trades on the market.
It is clear that executing a large buy order will push the price of an asset higher.
Similarly, executing a large sell order will push the price of an asset lower.
Arbitrage, or "arb," is a – theoretically – riskless trading strategy that involves buying and selling the same asset or risk position at differing prices. A trader or arbitrageur can do this because the asset is being offered for sale by one counterparty at a lower price than the price another counterparty is willing to pay for it.
Both arbitrageurs and high frequency traders operate in the space of small price differences.
Further, while high frequency trading obviously relies on speed, many forms of arbitrage are also dependent on speed.
However, there is a fundamental difference between the two activities.
Arbitrageurs take offsetting positions and profit from prices re-aligning.
Arbitrage is, at heart, a strategy that takes no risk, and profits from the reversion of prices.
High frequency traders, however, profit from taking a position and the price moving in the direction they want.
HFT is essentially a strategy that makes money by taking a risk position and profiting from a movement in the price of the relevant asset.
Arbitrageurs and high frequency traders both aim to profit from flows that are being hedged in the market, and are therefore very important to the way that market-makers operate.
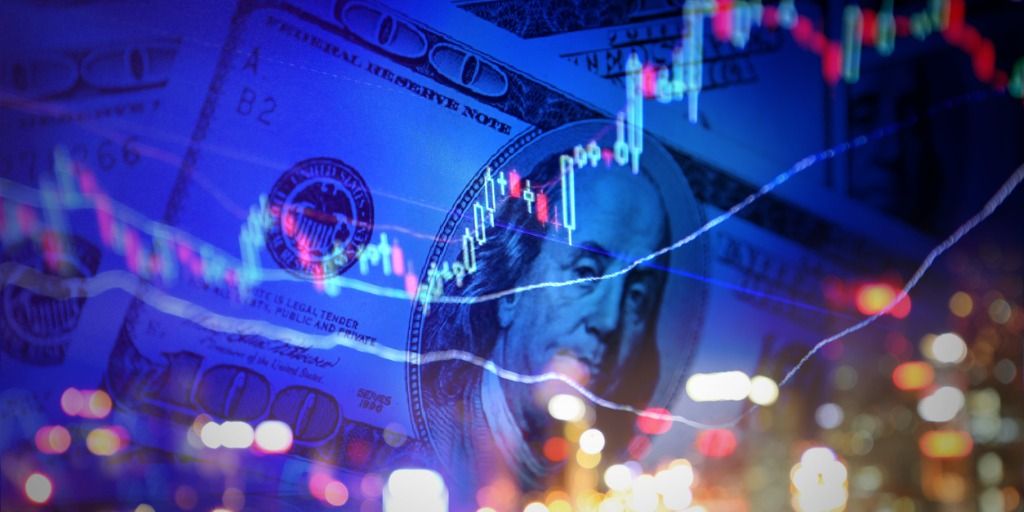
In the hunt for signal alpha, that is, predictive power that can be used to fuel position-taking in financial markets, quant teams use any tool and source any data they can find that might work.
Data providers, brokers, and aggregators have jumped on board too, and the industry is awash with "alternative" data.
Expert data is typically much more valuable than big data. Big data can verify a thesis, but rarely can it suggest one.
However, the biggest problem faced by machine learning – or other pure data-driven signals – is that most investor dollars are allocated by humans, who require a narrative to go with the risk-taking. Model-driven alpha does not provide this.
Perhaps this is the "holy grail" of machine learning – a true artificial intelligence that can explain the drivers of the machine learning signal to the human buyer of that signal.
Artificial intelligence and other quantitative techniques for signal generation are based on nontraditional data sources. These include so-called "big data" – data sets that are too large and complex to be processed using traditional database management applications.
Risk is a measure of the uncertainty in any future outcome. It can apply to:
- Quantifiable measures, such as P&L, provisions, balance sheet size, and quantity of regulatory capital.
- Less quantifiable aspects of a business, such as brand quality, reputation, and legal standing.
It is possible for the definition of risk to incorporate more than just losses or adverse changes in any of the above. For instance, an unexpected profit may be regarded as a risk, as it tells a firm something it doesn’t understand about its business.
This leads to a truer definition of what risk management is all about.
Risk management is a technique for verifying the understanding of a business against the reality experienced day-to-day when running that business. (Note, here, that the ''understanding'' of a business may be expressed in a spreadsheet model or risk report, or may even just reside in someone's head).
This is a fundamentally more impactful definition of risk management than just measuring and reporting numbers. When integrated into management decision-making, risk management serves a very important purpose beyond simply complying with reporting requirements.
Having said all that, quantitative techniques are an important aspect of risk management. A business has a model that by definition (it's a model) is simpler than the business, but captures its main points. It is vital that the model captures the salient characteristics of the business so that it has some predictive power when making resource allocation decisions.
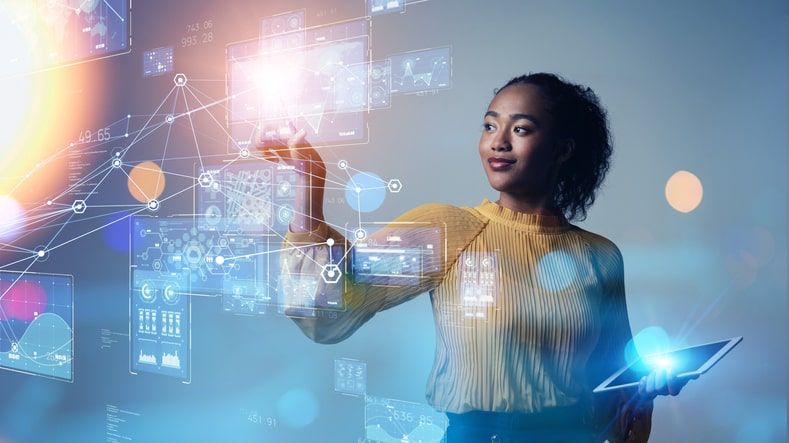
Constructing a portfolio involves an assessment of the risk and return of portfolio assets. One metric to compare the risk per unit of return on an asset is the Sharpe ratio.
Modern portfolio theory (MPT) shows how diversification can lead to the elimination of the risk specific to individual assets if the correlation between these assets is low or negative. This allows the investor or portfolio manager to focus on a desired level of systematic or general market risk.